
The Network Characteristic Analysis of Research Projects on International Research Cooperation
Abstract
In this study, the network analysis of researchers, institutions, and research principal agent was conducted to understand structure characteristics of international cooperation research project implemented from 1997 to 2018. The network of researchers and institutions were decentralized structure. On the other hands, the network of research principal agent was centralized structure. The Soul National University is the leading organization of international cooperation research project. In terms of research principal agent, corporation is the leading principal agent. In additions, the results of the network centroid analysis of the researchers and institutions were correlated with the research funds. As a result, it was confirmed that the network centroid of research organization was linearly related to research funds.
Keywords:
International Research Cooperation, Research Project, Research Report, Network, Centrality Analysis, Weighted Network, Linear Regression1. Introduction
Researchers from all over the world working in various fields conduct joint research projects to share knowledge, technology, and infrastructure. These joint research efforts are a collaborative link for the innovation and diffusion of knowledge and technology: moreover, international research cooperation is the process of acquiring new skills and know-how (Yang & Choi, 2008). Namely, international research cooperation is an important factor in the development of technology and securing competitiveness as it enables exchange of knowledge among cooperative researchers. Thus, international joint researches have become an important concept for scientific and technological innovation. Through these joint researches, we can identify the overall approach to measure interaction among researchers or countries to establish innovative competency in science and technology.
Although we could use citations from related academic papers that created through the international joint research to identify the types of shared technologies and cooperative relationship, there are limitations for identifying the extent of contribution or active roles in conducting researches. Therefore, in order to assess the extent of cooperation and level of research activities of those professionals in each country, a network analysis should be performed on the authors who jointly conduct the study for international academic journals. Various network analyses were conducted for authors of academic journals published within Korea through the international joint research (Lee, 2014; Jang, 2015). However, if the scope of analysis is limited to only academic journals, it will be hard to understand trends in all fields because the scope is narrowed down to certain fields or academic journals: there will be a need for conducting a research for international joint research network in various fields.
Therefore, this study conducted a network analysis of international joint research projects in various academic fields in Korea based on the database (DB) that the International Research Cooperation Information Center had established. We tried to identify the association attributes of international joint researches by categorizing and analyzing cooperation links by researchers, institutions, and research principal agent based on 2,500 international joint research projects from 2003 to 2018. In addition, we tried to identify the correlation between the centrality and research fund by comparing the network analysis results. In this study, we tried to derive various interpretations by analyzing the structural or cluster characteristics of the network; relying on the network analysis alone makes us hard to identify structural characteristics precisely, we also employed another analysis method such as machine learning. In other words, we could analyze the research activities of researchers in a various fields and could have visual identification of research team networks in respective fields.
This study is meaningful in identifying the current status and trends of Korea's international research cooperation; moreover, it is expected to contribute to the expansion of international research cooperation projects and vitalization of international joint research in Korea in the future.
2. Literature Review
This study used data from the International Research Cooperation Information Center's database and identified the network characteristics utilizing the Korean reports on international research cooperation subject area. We divided the relevant previous studies into three sectors. We examined the analysis of collaborative research networks in the library and information science (LIS) field, the analysis of collaborative research networks in other than LIS fields, and the correlation analysis by applying collaborative research networks method.
2.1 Co-author network Analysis in the Library and Information Science Field
The researchers who conducted the research most actively in relation to analysis of co-author networks in the LIS field were Lee Jae-yun and Lee Soo-sang.
First, Lee (2006a) suggested new measures such as nearest centrality, mean association, mean profile association, and triangle betweenness centrality to analyze the centrality of bibliometrical data as he mentioned that the recent trends in attempts to introduce a new method of centrality analysis, one of the tools of social network analysis, into a bibliometrics study, within the field of LIS. In the same year, Lee (2006b) presented a variety of network formation methods and characteristics that visually expressed bibliometrics data to make active use of different network formation in the related research that identified the intellectual structure. Afterwards, Lee (2014) conducted a comparative analysis on the centrality indices used to analyze the joint research network. Consequently, in order to measure both collaboration range and strength in a collaboration research network, weighted network centrality indices should be used; to identify global centrality, triangle betweenness centrality should be used; and to verify regional centrality, the SSR index should be applied. Recently, Lee (2016) verified the link between the co-author network’s centrality and research performance based on journals published in nine academic journals in the Korean LIS field from 2004 to 2013. Analyses showed that the relationship between centrality and research performance had a statistically significant positive correlation. However, another analysis showed that there was no correlation if the scope of analysis was limited to the top 30 authors.
Lee (2010) is a researcher who conducted the most active research on network analysis in the field of LIS; Lee conducted preliminary researches on the analysis of co-author network, a type of joint network, based on academic papers that were published in four Korean core academic journals from 2000 to 2009. Research result has shown that a Pareto’s rule could be applied to the number of papers submitted: the authors with high number of submitted paper also produce high number of joint work with others. Clearly, there are correlation between the academic impact indicators and authors with high in number of submitted paper. The following year, Lee (2011a) used the same data as the studies conducted in 2010 to identify the attributes of a co-author network based on the frequency of the four major journals in the field of LIS. As a result, various network structures had been created according to the frequency of collaborative works. The authors, of twenty-one pairs of co-author networks in total, were more active in joint research group with the frequency of more than five joint studies. The association of their research topic was found to be apparent in papers that were published in pairs of co-authors.
In addition to the research conducted by the above two researchers, Lee and Woo (2015) studied the statistics and co-author networks that were published in eight Taiwanese LIS journals from 2014 to 2015. Their centrality analysis results verified that the collaborative works’ range and strength were higher for the major researchers, led by professors at the National University of Taiwan. Kim and Lee (2016) conducted a research on the co-author network and reference network for information science journals; then, they identified the knowledge circulation structure in the fields of information science at home and abroad. Recently, Jeong (2017) analyzed collaboration patterns in four leading journals of LIS in Korea and found that the most actively collaborated research institutions in the network were Konkuk University and Dongwon University.
2.2 Co-author network Analysis in Other Fields
There are ongoing researches to identify cooperative relationship among authors through network analysis in various academic fields other than LIS. The network analysis for collaborative authors was conducted in two main forms. The first is to construct a network based on whether the authors of each paper collaborated with the authors of the other papers and identify the structural characteristics of the network (Kim et al, 2007). The second is to take a closer look at the impact of each node that consists of the network (Lee, 2011b). It is possible to identify the changes in network structure over time (Yang & Choi, 2008). Lee and Choi (2010) conducted a network analysis of the authors' network in the domestic convergence technology field to identify research cooperation relationships among authors. They also identified researchers who play the leaders in the research of convergence technologies based on the centrality values in co-author network analysis.
Also, Lee et al. (2011) conducted the co-author network analysis for papers published by the Korean Statistical Society from 2000 to 2010. They extracted the most closely connected subnetworks and conducted a degree centrality analysis, a closeness centrality analysis, and a between centrality analysis. Moreover, they performed a network analysis only for 2000 and 2010 to identify how the donor structure had changed over time. Leem (2012) derived the centrality values through the co-author network analysis on the journals of Logos Management Research, and identified the effects of these values on the research productivity of the authors.
Later, Jang (2015) conducted the co-author network analysis on international journals published by the National Cancer Center from 2000 to 2010. She verified the differences in researchers’ roles by conducting comparative analyses of the degree centrality and betweenness centrality values. She also analyzed trends in research activities of the most active co-authors by comparing the slope values of co-authors’ degree centrality. Furthermore, she analyzed the roles of authors more precisely by weighting their contribution.
Recently, Park, Park and Kang (2017) established a co-author network for research papers on leisure recreation fields. Unlike other previous studies, they took a different approach by applying weighted values depending on the number of authors in the published paper and their roles. They identified the structural characteristics of the network and the role of the major authors by performing the degree centrality, closeness centrality, betweenness centrality analysis.
2.3 Correlation Analysis using Network Analysis Results
It is possible to explain the interconnected nature of the network, which shows the cooperative relationship between components, by taking a quantitative approach with the centrality indicator. Based on this approach, it is possible to obtain statistically significant results by comparing and analyzing relationships with other characteristics.
Lee et al. (2011) conducted a centrality analysis through network analysis, and then applied logistic regression analysis to identify changes in collaborative research among researchers within the same agency.
Choi and Gim (2015) recently conducted the co-author network analysis based on the papers published by the Journal of Neuropsychiatry from 2009 to 2013. They applied the goodness-of-fit test to determine whether the co-author network shows a power law distribution. In addition, Poisson regression was performed to see the correlation between the h-index which corresponds to the research performance and the various independent variables which are the results of the network analysis: degree centrality, closeness centrality, betweenness centrality, and eigenvector centrality. Also, some researchers used nationality information from co-authors of the international psychiatry journal to identify the network structure between countries. To derive the results they used the network centrality analysis, the higher centrality indicators, and the total number of international joint research papers.
3. Methods
3.1 Data Acquisition and Analysis
In this study, network analysis was performed on the international joint research projects, which conducted research with foreign research institutions, among the national research programs from 1997 to 2018. The data in this study were based on research programs of National Science and Technology Knowledge Information Service (NTIS), and were collected through the International Research Cooperation Information Center. The International Research Cooperation Center's database contains a total of 2,500 data on joint research projects, each project contains information such as project serial numbers, research period, program name, project name, research management agent, principal research agent, and project organization, principal investigator, researchers, joint research agent, stages of research and development, scientific technology standard classification, applications, 6T related technologies, and research funding, etc. We extracted the program name, project name, principal agent, institution, principal investigator, researcher, joint research agent, scientific technology standard classification, and research funding information from 2,500 projects built in the International Research Cooperation Center database and used for network analysis.
In this study, two main network analyses were conducted to identify the network characteristics of the international research cooperation projects. Network analysis was conducted for researchers, institutions, and another network analysis on subject area, and the preprocessing process was as follows.
First, to perform network analysis for the researchers and institutions, we went through a preprocessing process such as extracting and rearranging the desired data from the database. Priority in the network analysis of participating researchers, including the lead researcher and the joint researcher, is the preprocess stage of distinguishing two different persons with the same name. However, in the case of research projects, the field and English names of participating researchers cannot be identified. In this study, we were able to complete the preprocess stage with the information from the institutions to distinguish the names of the researchers. In addition, preprocess should be preceded when performing network analysis to principal agent, including host institution or joint institution. In this study, we achieved the preprocess for principal agent by excluding academic cooperation or campus names but extracting university names.
Second, we identified networks by subject area of international joint research projects; then, we analyzed them in engineering, medical fields, natural sciences, and humanities. However, there are research projects do not have information on the subject area in the International Research Cooperation Information Center’s database. We used ‘classification of science and technology standards' and 'academic research area classification' by the Korea Research Foundation instead.
Several researches are underway to identify the cooperation structure of researchers through network analysis. A network is a set of connections between nodes and lines. The connectivity between the two nodes will vary depending on the topic of interest. Network analysis can be divided into two categories depending on complexity. The first is a one-mode network that expresses the relationship among members within a group. The other is a two-mode network that represents the relationship among all members within a large number of groups. The strength of the connection can be set differently by weighting the number or importance of nodes. In addition, depending on the subject forming the network, the direction of the connection may be specified.
In this study, we constructed three networks by designating the researcher, institution, and research principal agent as nodes. The weights and directions of the connecting lines were not taken into account in the networks of the researcher and institutions of the joint research. Weight was taken into account in the study subject network because there are fewer types of components. To visualize the network, the Frucherman Reingold Algoreithm was used. Because the algorithm does not take the weights between nodes into account, it is able to calculate faster and is able to distribute the nodes evenly. To examine basic network characteristics, the total number of connecting lines, the average number of lines per node, diameter, and density were analyzed. Density indicates how close the connections between the nodes and it can be calculated as the ratio of the number of possible connections to the number of actual connections. It represents the higher the number of connections between different nodes, the higher the density value. It also represents the higher the density value, the larger the subnetwork size. The node's centrality is a quantitative indicator of how much influence the node represents within the network. Due to its relativity in calculation of each element, one should be careful in interpreting the analysis results. Because of this characteristic, the centrality value should be expressed by normalizing it. Degree centrality indicates how many different nodes in the network are connected to others. It represents that the higher the degree centrality, the more relationships it has with other nodes within the network, and the greater its regional influence. However, the perspective of influence may vary depending on the perspective of network analysis. In order to identify the elements that act as intermediaries within a network, the betweenness centrality analysis is required. The higher the betweenness centrality of the node, the more influential it possesses in controlling the flow of information within the network. Eigenvector centrality shows high value when a node is connected to the node with a high connection count and high centrality accordingly. The farther away these nodes are, the lower their eigenvector centrality values. Because the eigenvector centrality represents a relative value in nature, it becomes more sensitive in terms of the number of connections as the network gets smaller. In this study, we performed a degree centrality, betweenness centrality, and eigenvector centrality on the researchers, institutions, and principal research agent’s network.
In this study, we performed a linear regression analysis to understand the correlation between the research fund and the degree centrality, betweenness centrality, and eigenvector centrality of the principal research agent and the conducting institutions’ network. For this purpose, we chose to analyze the researchers and institutions of the international joint research projects, which have relatively large number of nodes in the network. In the case of the researchers, we considered the research funding was allocated to the research director; we only utilized the director’s network centrality results. In the next step, there is no information available on the research funding allocated to the joint agent; we considered the research funding was all allocated to the principal agent.
3.2 Research Questions
In this study, we have identified the cooperative structure of the international joint research project carried out in Korea by conducting a network analysis of the subject areas of the researchers, institutions, and principle research agent. We tested the correlation between the leading elements of international cooperation research and the research funding. We established three research questions to identify those problems.
RQ 1: Is the international joint research network a distributed structure?
RQ 2: What factors are responsible for leading and mediating the international joint research project?
RQ 3: How does the network's centrality analysis result correlate with research funding?
4. Results
We performed a network analysis by extracting the researchers, institutions, and principal agent from the international joint research project database. We were able to identify the following by analyzing the cooperation structure among the subjects for analysis:
First is the density of connectivity between network components. In the researchers’ network, it is difficult to connect with researchers from other agents because they are usually connected to researchers from the same agent. However, in the agent’s network it is possible to expect close connections because there are participating institutions that conduct joint studies.
The second is to find the factors driving the international joint research project among network nodes. We were able to identify the components that make up the network through the analysis of centrality. In the case of principal agent, network analysis was carried out by segment. Therefore, it was possible to identify the main actors who lead the international joint research project by subject area.
Third, it was to find elements that act as intermediaries so that nodes can connect within a network.
Finally, it was to identify the correlation between the results of the network's centrality analysis and the research funds. From the three network analysis results, we tried to identify the subjects for analysis that are closest to the research funds.
4.1 International Joint Research Project Researcher Network Analysis
The visual representation of the network, which consists of 2,256 researchers participating in the international joint research project, is as shown in Fig. 1. If we look at visualized networks, the more links they have with researchers, the larger the node size and the greener it becomes. The most frequently linked ID 15, 63 and 41 researchers were expanded to visualize. The thicker the connection line, the more common studies have been performed. The enlarged picture confirmed that the researchers associated with the principal investigator's corresponding IDs 15, 63 and 41 are not connected to the research teams performing other research projects.
Table 1 shows the result of network statistics. The total number of connections is 2,149 and the joint researchers have an average of 1.95 relationships. Thirty-five connections are required to connect the two most remote researchers in the network. The network density is very low at 0.001. They tend to form network with number of small groups that members do not have close connections.
Table 2 shows the ranking of the network centrality. ID 15, 63 and 968 researchers have the most active interactions with the international joint research project researchers. The researchers, ID 624, 2, and 626, show relatively high values of betweenness centrality, which means that they act as intermediaries that coordinate collaborative research activities. However, the overall value of the betweenness centrality appears to be very small, indicating that the network groups are isolated from each other. ID 15, 407, and 408 researchers are shown high in terms of eigenvector centrality. All three are conducting research at the same institutions. This can be seen as influenced by ID 15 researcher who marks the highest degree centrality value.
4.2 International Joint Research Agent Network Analysis
We visualized a total of 271 international joint research project agents into the network (Fig. 2). If we look at visualized networks, the larger the node size and the greener it becomes as an agent is connected to more agents. The connections of Seoul National University, Korea Institute of Energy Research(KIER), and Korea Electronics and Telecommunications Research Institute(ETRI), which are the most frequently connected with other agents, were enlarged to display. Same as the researcher network, the thicker the connection line, the more joint studies were conducted among the connected research agents. We can see that all three agents have carried out international joint research projects only once with other agents.
Table 3 shows the statistics of research agent network analysis. The total number of connections is 180 and the participating agents have an average of 1.328 relationships. Five connections are required to connect the two most remote research agents in the network. Network density is very low at 0.005, indicating that the network is denser than the researcher network, but that the network is distributed in smaller groups.
Table 4 shows the ranking of the centrality analysis result. Seoul National University, Korea Institute of Energy Research, and Korea Electronics and Telecommunications Research Institute, which appear in deep green color, are engaged in many interactions with other research agents in conducting international joint research. Seoul National University, in particular, has the highest overall centrality values. This indicates that Seoul National University is a very important factor in conducting the joint research project. Since all of the betweenness centrality values show less than 0.01, it cannot be considered as a linkage even though the value is relatively high. Foreign universities are ranked second and third in eigenvector centrality. This means that Korean research institutes are the preferred agents for conducting international joint research.
4.3 International Joint Research Project Principal Research Agent Network Analysis
We conducted a network analysis with research institutions such as the governmental institutes, corporations, universities, foreign research institutes, government-funded research institutes, and other institutions as each node. We tried to find out which research agents were leading the international joint research project by subject area. We placed the nodes that are well connected to other types of research agents in the center.
We visualized the international joint research project’s principal research agents of all subject areas as a network (Fig. 3). If we look at the visualized network, we can see that corporations and universities are actively carrying out joint international research projects. We can also see that corporations prefer universities over government-funded research institutes to conduct international joint research projects. We can see that corporations are linked to all research agents.
Table 5 shows the research agent network analysis statistics. The total number of connections is 16 and the agents have an average of 53 relationships. Two connections are required to connect the two most remote research agents in the network. Density is very high at 1.067, indicating that all six nodes are tightly connected.
Table 6 shows the ranking of the centrality analysis results. We can see that the corporation with the largest node also has the most frequent interactions with other research agents in conducting international joint researches. In particular, corporations also have the highest value for the betweenness centrality and eigenvector centrality. This means that corporations have the greatest influence in carrying out the domestic joint research project.
Network representations of the agent of international joint research projects in the engineering field show that corporations and universities are conducting international joint research projects very actively. We can also see that corporations prefer universities to government-funded research institutes to conduct international joint research projects in engineering. We can see that corporations are linked to all research agents.
Table 7 shows the research agent network analysis statistic. The total number of connections is 12 and the research agents have an average of 39.33 relationships. Two connections are required to connect the two most remote research agents in the network. The density is very high at 0.8 which indicates that all six nodes are tightly connected.
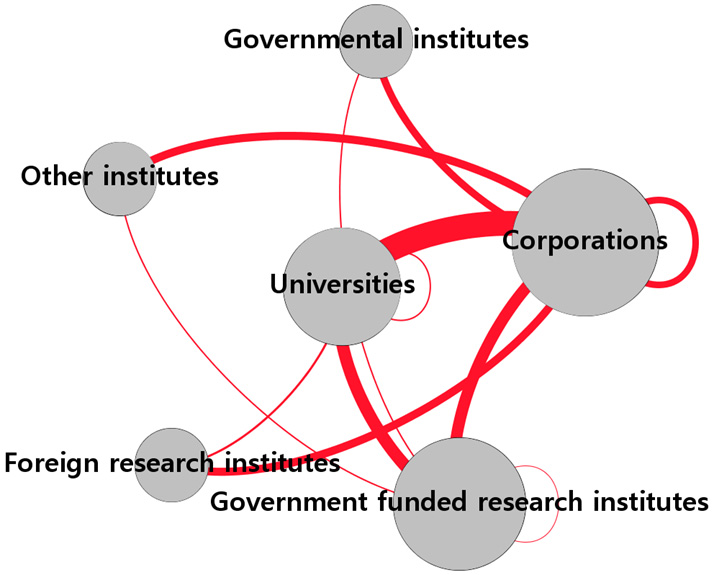
Principal research agent network analysis for international joint research project in engineering field
Table 8 shows the ranking of the centrality analysis results. We can see that the corporations have the largest nodes; and they have high frequency in interaction with other research agent in conducting international joint research in engineering field. In particular, corporations also have the highest value for the betweenness centrality and eigenvector centrality values. In contrast to all fields, the corporations’ betweenness centrality is significantly higher than that of other principal research agents. Corporations are the most active and influential actors in conducting international joint research projects in engineering.
We visualized the international joint research projects in medicine, natural science, and humanities fields were presented as network. The result of analysis of visualized networks in the field of medicine shows that the results are shown in Fig. 5. We can see that corporations are taking the lead in the international joint research project. Corporations are linked to all research subjects.
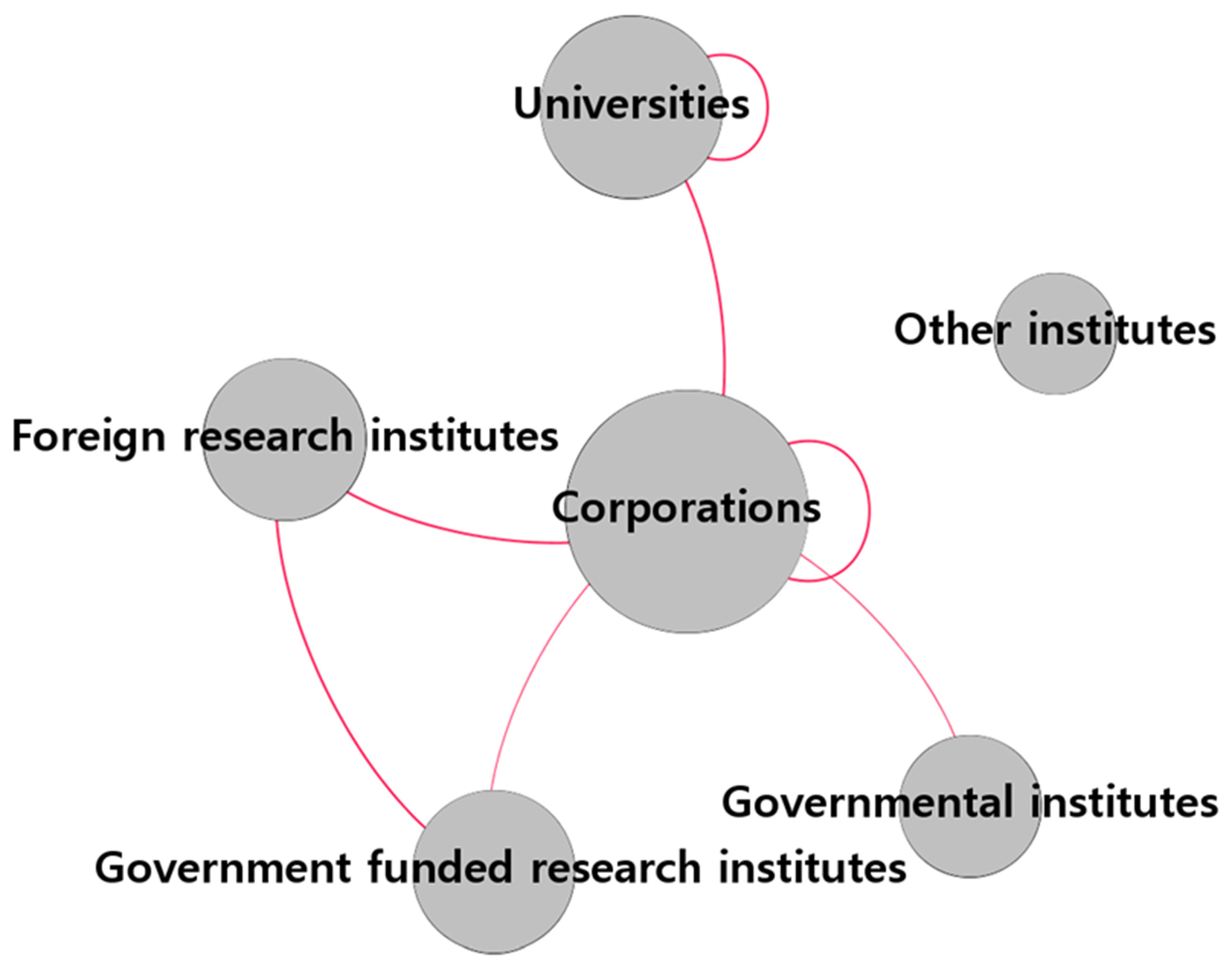
Principal research agent network analysis for international joint research project in medicine field
Table 9 shows the analysis statistics of the research agent network in the field of medicine. The total number of connections is seven, and the research agentshave an average of four relationships. Two connections are required to connect the two most remote research agents in the network. Density is low at 0.467 because there are no factors associated with other study subjects.
Table 10 shows the ranking of the centrality analysis. In this case, corporations also have the largest nodes and it shows most frequent interactions with other research agents in conducting international joint research in the field of medicine. In particular, corporations also have the highest value for the betweenness centrality and eigenvector centrality values. Corporations are the most active and influential actors in conducting international joint research projects in the field of medicine.
Based on the visualized network of natural sciences, Fig. 6 shows that universities are taking the lead in international joint research projects.
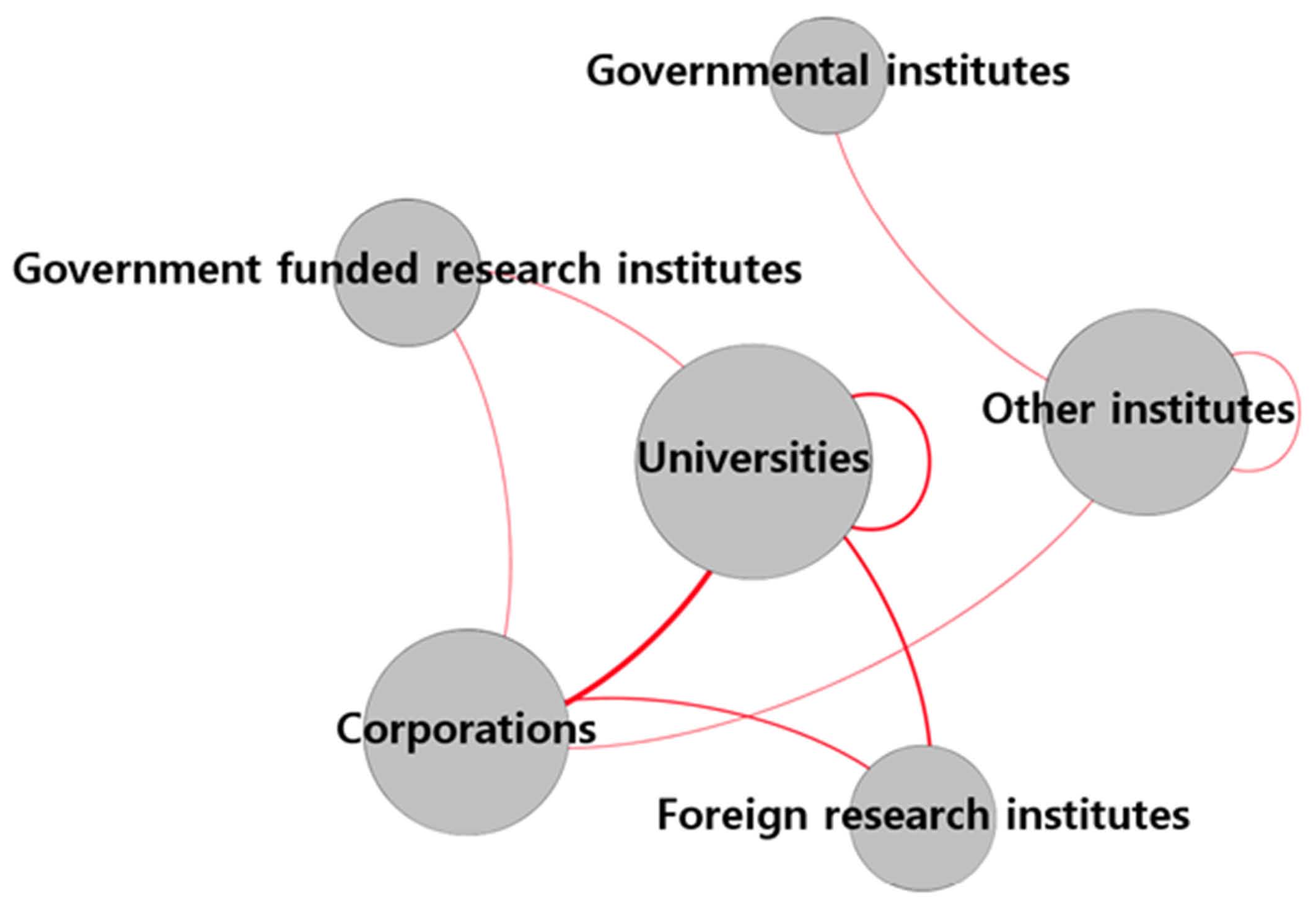
Principal research agent network analysis for international joint research project in natural science field
Table 11 shows the research agent network analysis statistics in natural sciences field. The total number of connections is nine, and the research agents have an average of six relationships. Three connections are required to connect the two most remote research agents in the network. Density is high at 0.6 and it is not such a close network but all components are connected.
Table 12 shows the rank of the centrality analysis result. We can see that the universities have the largest node, and it shows most frequent interactions with other research agents in conducting international joint research in the field of natural science. The corporations have a much higher value in terms of betweenness centrality than other factors. In terms of the value of eigenvector centrality, universities show higher values than corporations, but there is no significant difference. Therefore, in the field of natural science, we can say that the universities and corporations are leading international joint research projects.
If you look at Fig. 7 as a result of visualized network in the literature field, the governmental/public research institute, the government funded institutes, and other research agents do not carry out any international joint research projects.
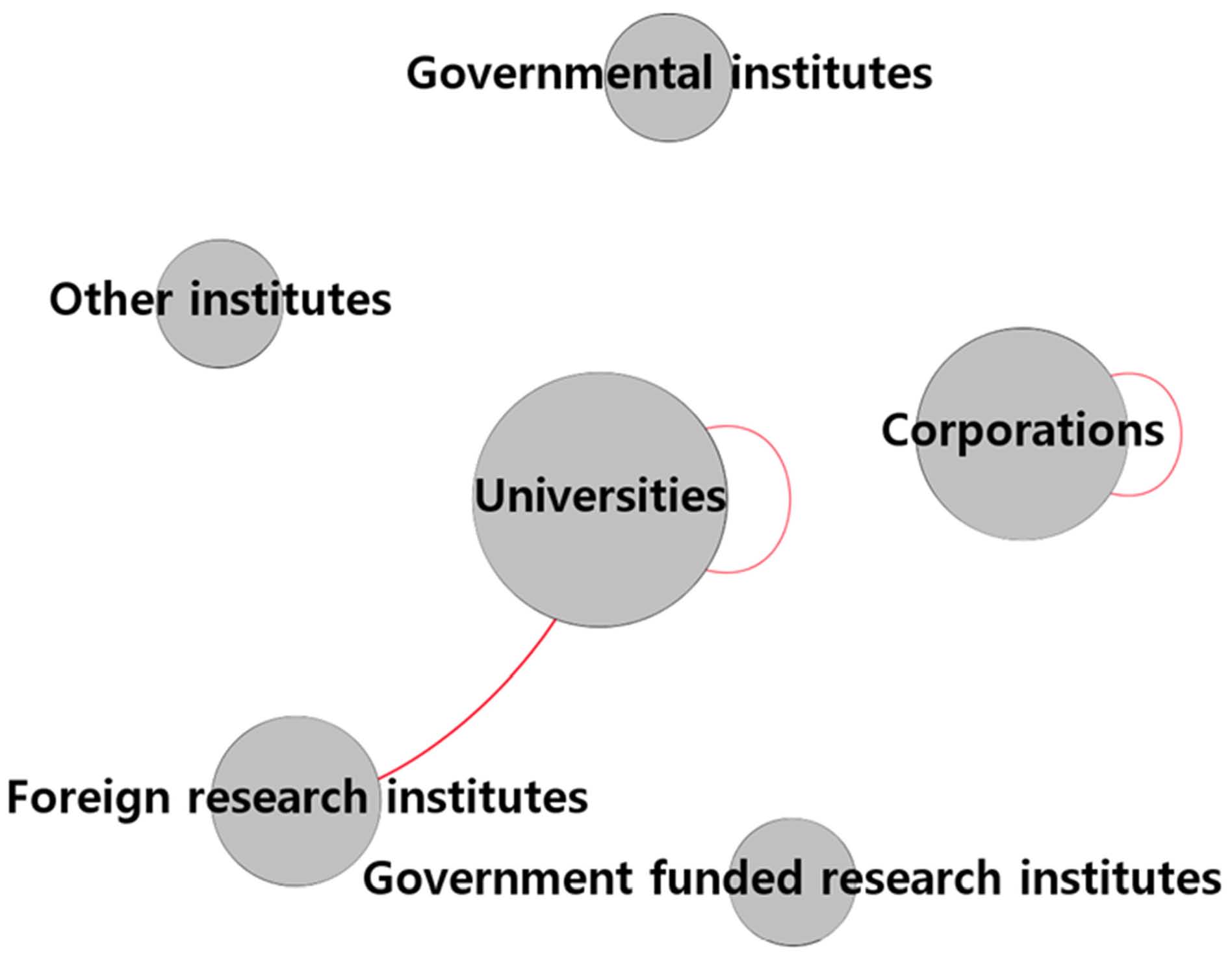
Principal research agent network analysis for international joint research project in humanities field
Table 13 shows a network analysis statistic for the humanities field. The density appears to be very low at 0.2. This is due to the universities, foreign research institutes, research agents are characterized by their lack of connectivity to other nodes. Table 14 shows the ranking of the centrality analysis results. Since there are no elements associated with more than two nodes, the mediated betweenness centrality as zero. The universities have the highest degree centrality and eigenvector centrality. Universities are the leader of international joint research in the humanities field.
4.4 Correlation Analysis on Network Centrality and Research Funds
Table 15 summarizes only the top 10 elements among the principal investigators of international joint research project in order of high research fund. The research funds were expressed in one million won. Researcher ID 106 has the highest allocation of research funds; however, there are only six researchers working jointly. In addition, his (her) betweenness centrality and eigenvector centrality value do not rank in the top 10. In the case of ID 956 and ID 1287 researchers, there are only two participating researchers carrying out the joint study. It goes the same as ID 106 researcher, their betweenness centrality and eigenvector centrality do not rank in the top 10.
Table 16 shows the results of a linear regression analysis after setting the network centrality of the international joint research’s principal investigator as an independent variable and setting the research funds as a dependent variable. The R2 values corresponding to the slope, y-intercept, and coefficient of determination are illustrated. The degree centrality, betweenness centrality, and eigenvector centrality all have a coefficient of determination value of less than 0.1. This indicates that the center value from the participating researcher's network analysis is irrelevant to the research funds.
Table 17 summarizes only the top 10 elements among the agents of international joint research project, in order of high research funds. The degree centrality analysis shows six of the top 10 research agents are included. The betweenness centrality analysis also includes five of the top 10 research agents.
However, eigenvector centrality analysis shows that only Seoul National University and the Korea Electronics and Telecommunications Research Institute are included among the top 10 institutions.
Table 18 shows the results of a linear regression analysis after setting the network centrality of the international community research institution as an independent variable and setting the research funds as a dependent variable. The degree centrality of the research agent indicates that the coefficient of determination value is greater than 0.6. The y-intercept has smaller value than zero but has a degree centrality value of 1. In other words, if there is one agent conducting a joint study, the predicted research funds will be greater than zero.
This indicates that the linear regression formula has been properly constructed and that the degree centrality of the research agent is directly proportional to the research funds. For the betweenness centrality and eigenvector centrality of the research agent, the coefficient of determination is greater than 0.4 but less than 0.5. Considering the slopes being all positive, the correlation coefficient value corresponding to the square root of the coefficient of determination is less than 0.7. Although the betweenness centrality and eigenvector centrality of the research agent represent a linear relationship with the research funds, we cannot say to it has a close relationship as the degree centrality.
5. Discussion
In this study, we performed a network analysis on the participating researchers, institutions, and principal research agents of the international joint research projects. We also performed the centrality analyses to quantitatively identify the network structure.
In the first study, we tried to identify whether a close connection exists in the international joint research project network. In the case of the participatory researcher network, there is little link among the researchers of other international joint research projects. It also indicates a very low density value at 0.001. In summary, we find that the participating researcher network is not closely connected: that is, it shows a distributed network structure. In the case of an institution network, connections to other projects appear relatively large, unlike the participating researcher network. However, Seoul National University, which has the highest degree centrality, is less than 5 percent of the total number of nodes. This indicates that different components form small groups. In addition, the density value is at 0.005, which is higher than the participating researcher network, but still represents a very small value. Therefore, institution network also has a distributed network structure that is not closely connected.
On the other hand, network analysis was carried out for research agents by subject area. In a principal research agent network of overall fields, all elements are connected as a single network. The network density value is very high at 1.067; therefore, it is not a distributed network structure. In the principal research agent network in engineering field, the density value is very high at 0.8, and all elements are connected as a single network structure. Therefore, it is not a distributed network structure. In a network of research agents in the field of medicine, other factors consist of a single network except for miscellaneous factors. A density value of 0.467 is not a high value, but it is not a distributed network structure because five elements are interlinked together. In the field of natural science, all six elements form a single network, and the density is high at 0.6. Therefore, it is not a distributed network structure.
In the second study we tried to identify the factors leading the international joint research project and the elements acting as intermediaries. In the case of the participating researcher network, there is a researcher that ranked first in both degree centrality and eigenvector centrality. However, the researcher is not connected to other researchers but to researchers within the same institution. Also, the factors of high betweenness centrality do not connect with other project researchers. Therefore, there are no factors in the network of the participating researchers that lead the international joint research project and act as intermediaries. In the institution network, Seoul National University is ranked first in degree centrality, betweenness centrality, and eigenvector centrality; it showed very high level of performance compared to other factors. It is also linked to other institutions that carry out international joint research projects. Therefore, Seoul National University plays the leading and intermediary role in the international joint research project. In a network of research agents in all fields, corporations show high importance of degree centrality, betweenness centrality, and eigenvector centrality. Corporations lead international joint research projects and act as intermediaries regardless of subject field. Similarly, in the field of engineering and natural sciences, corporations also exhibit high degree centrality, betweenness centrality, and eigenvector centrality. In the field of natural science, universities have the highest values for degree centrality and eigenvector centrality. However, the value of the betweenness centrality is much higher for the corporations. In the field of natural science, universities are leading international joint research projects, but corporations are responsible for mediating the researches. In the field of humanities, there is no intermediary role because the value of the betweenness centrality is all shown to be zero. Three out of four projects are linked to universities. Therefore, universities are the leading research agents in the humanities field.
In the third study, we tried to identify the correlation between the results of the network centrality analysis on the international joint research project and the research funds. We extracted and analyzed only principal investigators from the participating researchers. In terms of degree centrality, betweenness centrality, and eigenvector centrality analysis, the correlation coefficient with the research funds was less than 0.1. This indicates that the principal investigator’s network centrality value is nonlinear to the research funds. For the institution, the coefficient of determination of the betweenness centrality and eigenvector centrality was slightly less than 0.5. This means that although it is difficult to predict the research funds using the agency's betweenness centrality and eigenvector centrality, the two centrality figures represent linear relationships with the research funds. The degree centrality of the institution showed that the coefficient of determination value was greater than 0.6. In addition, the slope is positive and greater than 0.78 if converted to a correlation coefficient. This indicates that the degree centrality of the institution is closely connected linear relation to the research funds.
6. Conclusion and Suggestion
In this study, the network structure was quantitatively identified through the network analysis of the International joint research project researcher, institution, and principal research agent.
As a result of the study, the participating researcher network and the institution network represented the distributed structure, whereas the analysis of the research agents based on the all fields did not. This is because there are only six network components of the principal research agent.
In addition, there is no element to lead or act as an intermediary in the international joint research project because there is little connectivity between each research team in the participating researcher network. This indicates that researchers from various fields are working on a joint international research project on a variety of subjects.
However, if there is no interaction between the research teams, it means that each team's international joint research project is stopped and that its hard-established international human resources are destroyed. In the future, the international research cooperation information center must also establish a database of international human resources for future international joint research.
In addition, there are researchers who do administrative work, rather than actually conducting research. Therefore, if the weight of research results, such as papers or patents from each international joint project, can be assigned, the research team can quantitatively identify the role performed by the participating researchers and how actively each research team has undertaken the project.
Seoul National University leads the international joint research project and act as an intermediary. However, only about 5 percent of the institutions are directly connected to SNU. Seoul National University is mostly connected to other universities, and there are very few connections among the various types of research agents, such as corporations or other research agents.
Combining the networks of the participatory researcher and the institutions, we can see that many research teams are working on a variety of international joint research projects, but each research team is working on closed research projects. Care should be taken in interpreting the institution network because weight and direction were not taken into account to the principal agent and participatory agent that are responsible for the international joint research project, which we did not take into account the contribution of the principal agent. Therefore, it is necessary to assign weights for future research depending on the role of the research agents conducting the international joint research project.
Corporations take lead and play an intermediary role in the research agent network in all fields, engineering fields, and medicine fields for in the international joint research project. This might be caused of failure to classify corporations by the size: large, medium, and small businesses. If we have taken further steps of detailed breakdown of firms into network components, it may result in universities are leading international joint research projects.
Also, care should be taken in interpreting the results of network centrality for corporations because it represents more than 100 firms leading the international joint research project rather than a small number of firms. In the field of humanities and natural sciences, care should be taken in interpreting the results of network centrality analysis because it seems like universities are leading the international joint research projects; however, universities are carrying out fewer than 10 projects. The centrality analysis result of the participating researcher network and research fund exhibits a very low coefficient of determination. We can assume that labor expenditures are high in research funding. However, there may be cases of low research fund where expenditures are highly concentrated on purchasing research equipment or materials for experiments rather than labor costs; and there may be cases of very high research fund where expenditures are intended for building laboratory buildings or purchasing large equipment. A very high coefficient of determination would have been shown if we could analyze and compare the participatory researcher network centrality based on the agent’s labor expenditures. The degree centrality of the principal agent is very closely related to research funding. However, the linear equation inferred from this study may only be valid within the range of specific centrality values due to each institution interlinked with less than four other institutions. In addition, the joint agent’s research fund is also included in the principal agent’s research fund. If we could use the actual research fund then analyze entire international joint research project and principal agents, the network centrality of the institution may show not only the degree centrality but also the eigenvector centrality and high coefficient of determination.
If it becomes possible obtaining the accurate information about research fund in the future, there will be a need for designing a more precise prediction model and further researches reflecting the new model.
References
-
Choi, M., & Gim, M., (2015), The Characteristic Analysis of Researches Network for Journal of Korean Neuropsychiatric Association, Journal of Korean Neuropsychiatr Association, 54(4), p418-426.
[https://doi.org/10.4306/jknpa.2015.54.4.418]
-
Jang, H. L., (2015), Generation of Collaboration Network and Analysis of Researcher’s Role in National Cancer Center, Journal of the Korea Contents Association, 15(10), p387-399.
[https://doi.org/10.5392/jkca.2015.15.10.387]
- Jeong, Y. K., (2017), An Exploration of the Collaborative Topic Analysis Adopting a Spatial Approach in Korean LIS Researches, Journal of the Korean Society for Information Management, p19-22.
- Kim, Y. H., Yoon, J. R., Cho, H. S., & Kim, Y. J., (2007), Structure of Collaboration Network among Korean Scientists - ‘Small World’ and Position Effect, Korean Journal of Sociology, 41(4), p68-103.
-
Kim, E. J., & Lee, S. S., (2016), Analysis of Characteristics of Knowledge Structuralization in Domestic and International Information Science through Network Analysis, Journal of Social Science, 27(3), p53-77.
[https://doi.org/10.16881/jss.2016.07.27.3.53]
-
Lee, M. H., Park, M. R., Lee, H. J., & Jin, S. H., (2011), Analysis of Papers in the Korean Journal of Applied Statistics by Co-Author Networks Analysis, Korean Journal of Applied Statistics, 24(6), p1259-1270.
[https://doi.org/10.5351/kjas.2011.24.6.1259]
- Lee, S. S., (2010), A Preliminary Study on the Co-author Network Analysis of Korean Library & Information Science Research Community, Journal of Korean Library and Information Science Society, 41(2), p297-315.
- Lee, S. S., (2011a), A Analytical Study on the Properties of Coauthorship Network Based on the Co-author Frequency, Journal of Korean Library and Information Science Society, 42(2), p105-125.
- Lee, S. S., (2011b), Analytical Study on the Relationship between Centralities of Research Networks and Research Performances, Journal of Korean Library and Information Science Society, 44(3), p405-428.
-
Lee, Y. G., & Woo, Y. H., (2015), A Study on Analyzing Co-authorship Networks of Library and Information Science in Taiwan, Journal of the Korean Society for Information Management, 32(2), p167-192.
[https://doi.org/10.3743/kosim.2015.32.2.167]
-
Lee, J. Y., (2006a), Centrality Measures for Bibliometric Network Analysis, Journal of the Korean Society for Library and Information Science, 40(3), p191-214.
[https://doi.org/10.4275/kslis.2006.40.3.191]
-
Lee, J. Y., (2006b), A Study on the Network Generation Methods for Examining the Intellectual Structure of Knowledge Domain, Journal of the Korean Society for Library and Information Science, 40(2), p333-355.
[https://doi.org/10.4275/kslis.2006.40.2.333]
- Lee, J. Y., (2014), A Comparative Study on the Centrality Measures for Analyzing Collaboration Networks, Journal of the Korean Society for Information Management, 31(3), p153-179.
-
Lee, J. Y., (2016), Comparative Analysis on the Relationships between the Centralities in Co-authorship Networks and Research Performance Considering the Number of Co-authors, Journal of the Korean Society for Information Management, 33(3), p175-199.
[https://doi.org/10.3743/kosim.2016.33.4.175]
-
Lee, J. M., & Choi, M. S., (2010), Social Network Analysis on Interdisciplinary Collaboration of Convergence Technologies Specialists, Journal of the Korea Contents Association, 10(6), p415-428.
[https://doi.org/10.5392/jkca.2010.10.6.415]
- Leem, B. H., (2012), An Effect of Co-authorship Network on Research Performance: Focusing on Co-authoring of Logos Management Review, Logos Management Review, 10(1), p1-20.
-
Park, S. G., Park, K. W., & Kang, H. W., (2017), The Related Word Analysis on Korean Society of Leisure and Recreation Based on Text Mining and Network Analysis: Focused on KAHPERD, The Korean Journal of Physical Education, 56(3), p389-402.
[https://doi.org/10.23949/kjpe.2017.05.56.3.29]
- Yang, C. H., & Choi, C. H., (2008), The Study on the Research Cooperation between Korea and Taiwan, The Korean Association For Public Administration, p171-189.
Younghee Noh has an MA and PhD In Library and Information Science from Yonsei University, Seoul. She has published more than 50 books, including 3 books awarded as Outstanding Academic Books by Ministry of Culture, Sports and Tourism (Government) and more than 120 papers, including one selected as a Featured Article by the Informed Librarian Online in February 2012. She was listed in the Marquis Who’s Who in the World in 2012-2016 and Who’s Who in Science and Engineering in 2016-2017. She received research excellence awards from both Konkuk University (2009) and Konkuk University Alumni (2013) as well as recognition by “the award for Teaching Excellence” from Konkuk University in 2014. She received research excellence awards form ‘Korean Library and Information Science Society’ in 2014. One of the books she published in 2014, was selected as ‘Outstanding Academic Books’ by Ministry of Culture, Sports and Tourism in 2015. She received the Awards for Professional Excellence as Asia Library Leaders from Satija Research Foundation in Library and Information Science (India) in 2014. She has been a Chief Editor of World Research Journal of Library and Information Science in Mar 2013~ Feb 2016. Since 2004, she has been a Professor in the Department of Library and Information Science at Konkuk University, where she teaches courses in Metadata, Digital Libraries, Processing of Internet Information Resources, and Digital Contents.
Taeyoun Kim is a researcher in the Institute of Knowledge Content Development Technology. He earned a bachelor’s degree in Geo-environment system engineering from Hanyang University, South Korea and Ph.D in Petroleum Resource Technology from University of Science and Technology, South Korea. He is interested in big data analysis such as data mining from social media website, DB management, network analysis, Machine learning, and Deep learning. He has experience about massive data processing and making equations for calculating properties by parallel computing.
Rosa Chang is a researcher at the Institute of Knowledge Content Development and Technology. She works for the Tongwon University of Library and Data Science as an Instructor. She received her Ph.D. degree in Library and Information Science from Chungang University. Her research interests include Human Computer Interaction (HCI), Information Architecture (IA), Digital Library, Knowledge Sharing, Reading Education.